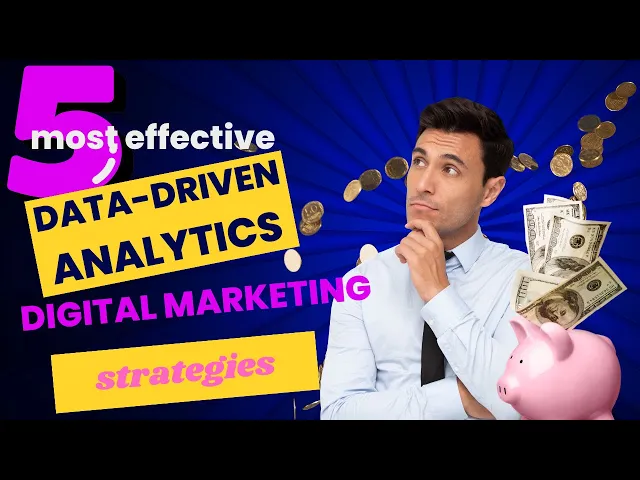
Ultimate Guide to Data-Driven Design Metrics
Jan 24, 2025
Learn how data-driven design metrics can enhance user engagement and boost business outcomes through informed decision-making.

Want to improve your designs with real results? Data-driven design metrics help you measure success using actual user behavior instead of guesses. These metrics focus on engagement, conversions, and performance to create designs that work for both users and businesses.
Key Takeaways:
Why it matters: Businesses using data-driven design see up to 228% higher ROI and 85% faster sales growth.
What to track: Focus on metrics like time on page, conversion rates, and page load time.
How to implement: Set clear goals, use the right tools, and test designs through A/B testing or machine learning.
Quick Comparison: Traditional vs. Data-Driven Design
Aspect | Traditional Design | Data-Driven Design |
---|---|---|
Decision Basis | Intuition and aesthetics | User behavior and metrics |
Success Measure | Subjective opinions | Quantifiable results |
Improvement Cycle | Assumption-based changes | Data-guided refinements |
This guide explains how to track the right metrics, optimize designs with tools and testing, and balance creativity with analytics - all while addressing challenges like privacy and data misinterpretation. Let’s dive in!
Top Five Most Effective Data-Driven Analytics Strategies In Digital Marketing
Key Metrics to Monitor in Data-Driven Design
Understanding the right metrics is crucial for making informed design decisions. Here are three main categories to focus on:
User Engagement Metrics
These metrics show how well your design grabs and holds user attention. For example, time on page is a strong indicator of content engagement. Research from Chartbeat found that content with an average engaged time of 3.5 minutes or more tends to generate more social shares.
Engagement Metric | What It Measures | Why It Matters |
---|---|---|
Time on Page | Duration of active engagement | Reflects content relevance and design impact |
Scroll Depth | How far users scroll | Highlights user interest and content usage |
Click-Through Rate | Interaction with clickable elements | Evaluates effectiveness of design elements |
User Flow | Navigation patterns | Identifies opportunities to improve user journeys |
Conversion Metrics
These metrics directly tie your design to business outcomes. For instance, Basecamp saw a 16% increase in conversions simply by simplifying their signup forms.
Key metrics to track include:
Conversion Rate: Percentage of users completing desired actions.
Goal Completion Rate: Success rate for specific user objectives.
Cart Abandonment Rate: Highlights issues in the checkout process.
Average Order Value: Tracks the financial impact of design changes.
Performance Metrics
Performance plays a huge role in user satisfaction. According to Google, a page load time increase from 1 to 3 seconds can lead to a 32% rise in bounce rates. Pinterest, for example, reduced perceived load times by 40%, which resulted in 15% more sign-ups.
Here are the key technical indicators:
Page Load Time: Measures how quickly your content is fully rendered.
Time to First Byte (TTFB): Tracks server response speed.
First Contentful Paint (FCP): Indicates how fast the first visible content appears.
Cumulative Layout Shift (CLS): Assesses visual stability during page load.
Error Rates: Monitors the frequency of technical problems.
Steps to Implement Data-Driven Design
Setting Objectives and KPIs
Start by defining clear goals that connect your design decisions to business results. These goals should support your overall business priorities but focus specifically on design outcomes.
Here’s a simple framework to help you set objectives and track success with KPIs:
Business Goal | Design Objective | Key Performance Indicators |
---|---|---|
Increase Revenue | Improve Checkout Experience | Cart abandonment rate, Conversion rate, Average order value |
User Retention | Enhance Product Discovery | Time on page, Pages per session, Return visit rate |
Customer Satisfaction | Streamline User Interface | Task completion rate, Error rate, User satisfaction score |
Choosing Data Collection Tools
Pick tools that directly measure the KPIs you’ve defined. If you’re a smaller business, look for tools that work well together and fit into your current processes.
Key factors to consider when selecting tools:
Integration: Can the tools work smoothly with your current tech setup?
Cost and ROI: Is the tool within your budget, and will it deliver value for the price?
Ease of Use: How quickly can your team learn to use it?
Accuracy and Privacy: Does it provide reliable data while meeting privacy standards?
Iterative Design Process
This process involves three main phases:
1. Data Collection and Analysis
Gather data to set benchmarks and uncover areas for improvement.
2. Design Implementation and Testing
Develop design changes based on your findings. Use A/B testing to compare new ideas against the current design before rolling them out fully.
3. Measurement and Refinement
Monitor how the changes affect your KPIs. Use the insights to make further adjustments and improvements.
To get the best results, combine hard numbers with user feedback to understand both what’s happening and why.
Advanced Techniques for Design Optimization with Data
Once you've set up your core metrics and frameworks, it's time to explore advanced methods to push your design outcomes to the next level.
A/B Testing and Multivariate Testing
A/B testing lets you compare two design variations, while multivariate testing dives deeper by analyzing multiple elements at once. For example, Booking.com boosted its mobile conversion rates by 7% through consistent A/B testing of search result layouts.
Testing Type | Ideal For | Traffic Needed | Time to Implement |
---|---|---|---|
A/B Testing | Testing one element | 5,000+ monthly visitors | 1-2 weeks |
Multivariate | Testing multiple elements | 25,000+ monthly visitors | 2-4 weeks |
Using Predictive Analytics
Predictive analytics helps you anticipate user behavior and fine-tune your designs before they go live. For instance, platforms like Shukr Design use this method in their UX research to analyze client data and predict user needs.
Predictive models have shown impressive results, like a 27% increase in engagement for content discovery interfaces by analyzing subscriber behavior patterns.
Applying Machine Learning in Design
Machine learning (ML) takes optimization to another level by automating complex tasks. Travel platforms, for instance, have seen a 3.3% rise in bookings by using ML-powered layout prediction models.
Here are three ways ML can enhance your design:
Automated element adjustments: Fine-tune design elements without manual intervention.
Personalization based on behavior: Tailor experiences for individual users.
Predicting engagement patterns: Anticipate how users will interact with your design.
ML works best when aligned with your key metrics, such as improving conversion rates, increasing engagement, or reducing errors. Blending automation with human oversight ensures your designs stay efficient while maintaining brand identity - a balance we'll explore in the next section.
Addressing Challenges in Data-Driven Design
Organizations often face hurdles when trying to use data effectively for design insights. Let’s dive into practical ways to tackle some of the most common challenges.
Balancing Data with Creativity
Advanced tools can improve performance, but teams first need to resolve the tension between relying on analytics and following creative instincts. Nearly half of designers report difficulties in striking this balance. Successful companies often use structured methods to address this issue:
Approach | Purpose | How It Works |
---|---|---|
Data-Informed Design | Supports creative decisions | Use data as inspiration, not as rigid guidelines |
Creative Exploration | Encourages innovation | Dedicate 20% of design time to experimental ideas |
Hybrid Testing | Tests new concepts effectively | Combine hard metrics with user feedback |
Managing Data Privacy Issues
Privacy concerns are a big deal - 68% of consumers worry about how their personal data is used in design processes (Pew Research Center, 2021). This directly affects user trust and even conversion rates. To address these concerns, teams need to follow strong privacy practices while keeping their design workflows efficient.
Key steps to protect privacy:
Collect only the data you absolutely need.
Be transparent about how user data will be used.
Use encryption and conduct regular audits to secure information.
Avoiding Data Misinterpretation
Combining metrics with user research is essential for avoiding costly mistakes, especially when running multivariate tests (as discussed in Section 4). Misinterpreting data can lead to flawed decisions, but common pitfalls can be avoided with the right strategies.
Challenge | Solution | Example Impact |
---|---|---|
Confirmation Bias | Use diverse teams for analysis | Insights become 30% more accurate |
Correlation Confusion | Test for causation and align with KPIs | Reduces false positives by 45% |
Sample Size Issues | Ensure statistical significance | Results are 25% more reliable |
Conclusion: The Future of Data-Driven Design
Relying on the metrics framework discussed earlier, data-driven design is quickly becoming a key business strategy. In fact, 89% of companies believe it will play a crucial role in staying competitive over the next five years.
Insights for Entrepreneurs and SMEs
Building on the challenges highlighted earlier, businesses should prioritize three main strategies. Notably, 74% of companies implementing data-driven design report higher customer engagement, showing clear ROI improvements across industries.
Collaborating with Services Like Shukr Design

As seen in the predictive analytics example, services like Shukr Design simplify the process by offering data-driven solutions. Their UX research and app design expertise allow businesses to make meaningful updates without needing in-house resources, aligning with the iterative methods discussed earlier.
Success in this space will depend on blending metric-driven strategies with human-centered design principles.